Translate this page into:
Transforming orthodontics with artificial intelligence: A comprehensive review
*Corresponding author: Sanika Mukund Thakur, Department of Orthodontics and Dentofacial Orthopedics, Ranjeet Deshmukh Dental College, Nagpur, Maharashtra, India. thakursanika2019@gmail.com
-
Received: ,
Accepted: ,
How to cite this article: Thakur SM, Shenoy U, Hazare A, Karia H, Khorgade P, Nandeshwar N, et al. Transforming orthodontics with artificial intelligence: A comprehensive review. J Adv Dental Pract Res. doi: 10.25259/JADPR_26_2024
Abstract
Orthodontics, the dental specialty dedicated to correcting misaligned teeth and enhancing dental aesthetics, has experienced notable progress with the incorporation of artificial intelligence (AI). This article offers an in-depth review of AI’s role in the field, emphasizing recent advancements, current uses, and potential future developments. By leveraging AI, orthodontics has seen significant improvements in diagnosis, treatment planning, and patient outcomes. The discussion also explores the promising directions AI technology may take in further revolutionizing orthodontic care. AI is being applied across multiple aspects of orthodontic practice, with notable applications in image analysis, treatment planning, and outcome prediction. AI-powered software can analyze dental images, including X-rays, panoramic radiographs, and intraoral scans, to detect and diagnose orthodontic conditions with high accuracy. Furthermore, AI algorithms can assist orthodontists in creating personalized treatment plans by simulating tooth movements, predicting treatment outcomes, and optimizing treatment sequences. These systems also support remote monitoring of patient progress, enhancing treatment adherence, and facilitating timely interventions. Looking ahead, the future of AI in orthodontics holds immense promise for further advancements and innovations. Continued research and development efforts are expected to refine existing AI algorithms and expand the scope of AI applications in orthodontic practice. Areas of future exploration include the integration of AI with emerging technologies, such as 3D printing and virtual reality, to enhance treatment customization and patient engagement. In conclusion, AI is poised to revolutionize orthodontic practice, offering unprecedented opportunities to enhance diagnosis, treatment planning, and patient care. By harnessing the transformative power of AI, the orthodontic community can advance the field, improve treatment outcomes, and ultimately enhance the quality of care for patients worldwide.
Keywords
Orthodontics
Artificial intelligence
Application
Technology
Diagnosis
Scope
INTRODUCTION
Artificial intelligence (AI) is the technology that enables machines to mimic human intelligence using smart algorithms. It involves creating intelligent machines through advanced computer programs. AI focuses on making computer systems understand and replicate human intelligence without being limited to biological methods. This research is more practical, emphasizing the application of AI rather than its theoretical aspects.[1]
This research is more of an application based than the theoretical aspect of AI. The ability to comprehend and mimic a skilled person, such as an orthodontist, to solve an optimization problem with processing power comparable to a standard computer system can be broadly described as an application. Using AI in orthodontics to treat misaligned teeth, the repetitive steps in diagnosis can be reduced. This combination of AI and orthodontics will help automate the process, making it more accurate and consistent than manual methods. It will also decrease the need for manual labor, which saves both time and money. Overall, AI can streamline orthodontic treatments.
In orthodontics, specifically, AI plays a crucial role in enhancing treatment efficiency, precision, and patient outcomes. From automating cephalometric analysis to customizing orthodontic appliances and predicting treatment outcomes, AI-driven solutions are transforming the way orthodontists diagnose, plan, and execute treatment.
TERMINOLOGIES
“Artificial” refers to something created by people or something that is not natural, and “intellect” refers to the capacity for comprehension or appropriate thought. “AI is basically the study of teaching your machine to mimic a human brain and its thinking capabilities” is another way to put it.[2] Machine learning, a part of AI, predicts outcomes using algorithms and data. Its main goal is to enable computers to learn from data so they can solve problems on their own, without human help. By analyzing patterns in the data, machine learning systems can make decisions and improve over time. This technology is used in many areas to create smarter and more efficient solutions [Figure 1].
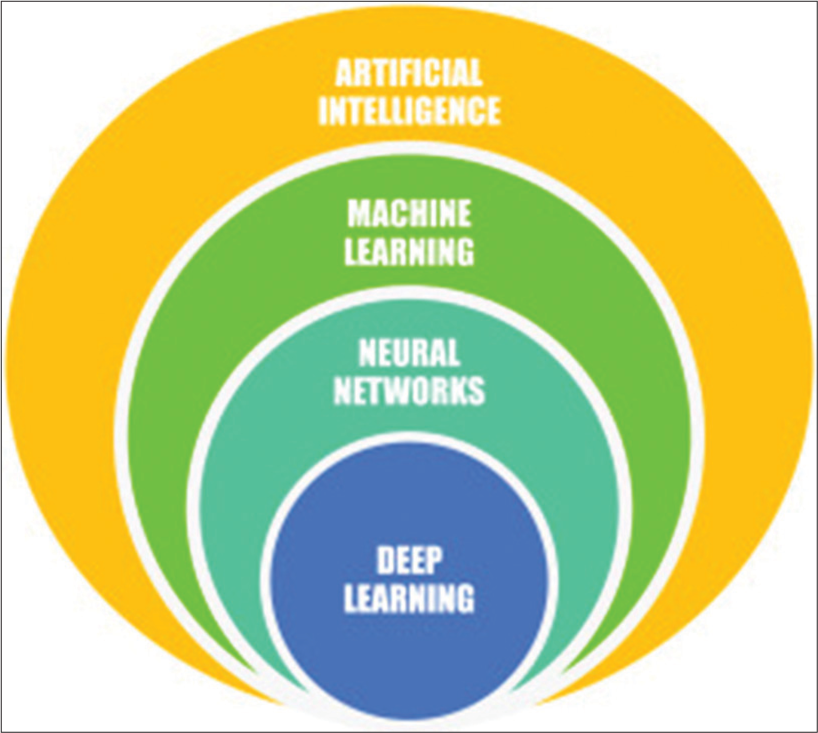
- Layers of artificial intelligence.
Machine learning can be divided into two groups
Supervised machine learning (ML)
Unsupervised ML.
Supervised learning
Supervised learning is a technique where training data and human feedback are used to teach an algorithm the relationship between inputs and outputs. When the output data is known in advance, it is used. After analyzing this data to identify the patterns, the system is able to make precise predictions about new data. This kind of learning aids the software in forecasting accurately using the patterns it has discovered.[3]
Unsupervised learning
This refers to a branch of machine learning where models are trained on unlabeled datasets and are then permitted to operate unsupervisedly on the data.
The benefits of unsupervised learning
Because labeled input data are unavailable for unsupervised learning, it is utilized for more complicated tasks than supervised learning
Compared to labeled data, unlabeled data is easier to get, making unsupervised learning the preferred method.
Disadvantages of unsupervised learning
Because unsupervised learning lacks a comparable output, it is inherently harder than supervised learning. Due to unlabeled input data and algorithms’ ignorance of the precise output, the outcome of an unsupervised learning algorithm may be less reliable.
Artificial neurons are used by neural networks, a particular kind of algorithm, to process data. Creating systems that can carry out tasks in a manner akin to the functioning of the human brain are the primary objective of neural network design. These networks use data analysis and machine learning to solve problems and make judgments. In many different applications, neural networks are utilized to simulate the processing and comprehension of the brain.
The fundamental components of artificial neural networks (ANNs) are node layers, which include an input layer, an output layer, and one or more hidden layers. Each artificial neuron, or node, has a weight and a threshold that connects it to other nodes. When a node’s output exceeds a set threshold, it activates and sends data to the next layer of the network. If it does not exceed the threshold, no data is passed onto the next layer [Figure 2].
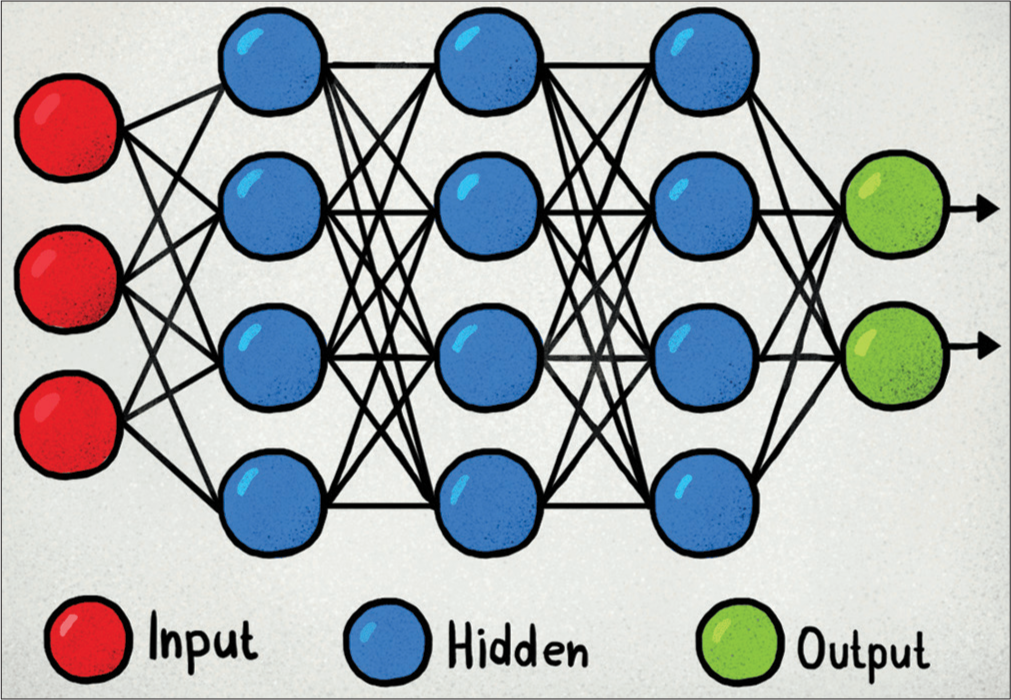
- Layers of the artificial neural network.
Neural networks require training data to learn and become more accurate over time. Once they are adjusted for precision, these learning algorithms are strong instruments in computer science and AI that enable us to rapidly categorize and group data. A biological neuron and a neuron in a neural are comparable. It takes in information from the other neurons, processes it, and then outputs something [Figure 3].

- Biological neuron.
AN ARTIFICIAL NEURON
In this case, Y denotes the neuron’s output, f(X) is the processing performed on the inputs, and X1 and X2 are the inputs to the artificial neurons. 3 layers make up a neural network [Figure 4]:

- Artificial neuron. X1, X2 are the inputs, f (x) processing centers, Y is the output.
Input layer
This layer comes first. The data will be received by this layer and then forwarded to the other network layers.
Hidden layer
The term “hidden layer” refers to the second kind of layer. Neural networks have exceptional performance and complexity due to the presence of hidden layers. They carry out several tasks concurrently.
Output layer
The output layer is the final category of layers. The solution or output of the issue is stored in the output layer.
One subfield of machine learning called “deep learning” uses a deep neural network with several computational layers to interpret incoming data. Deep learning aims to improve feature recognition by using a neural network to automatically identify patterns.
GOALS OF AI
Enhance problem-solving skills
Integrate knowledge representation
Enable effective planning
Support ongoing learning
Foster social intelligence
Stimulate creativity
Attain general intelligence
Encourage collaboration between humans and AI.
AI REVOLUTIONIZES DENTISTRY
Education: Intelligent tutoring systems simulate clinical scenarios, accelerating student skill development[4]
Patient Management: Virtual dental assistants streamline administrative tasks with accuracy and efficiency[4]
Treatment and Diagnosis: AI aids in disease diagnosis, treatment planning, and prognosis prediction
Radiology: AI enhances diagnostic procedures, improving speed and accuracy in imaging
Surgery: Robotic surgery improves precision and safety in oral and maxillofacial surgery 6. Prosthodontics: AI designs cosmetic prostheses based on patient preferences and anthropological calculations
Endodontics: AI enhances treatment planning and disease diagnosis, detecting subtle changes[5]
Forensic Dentistry: AI assists in identifying biological age, gender, and analyzing bite marks[6]
Innovations: AI-powered dental chairs with voice-command capabilities enhance patient comfort and monitoring
Future Prospects: Bioprinting with AI offers potential for tissue reconstruction in dentistry [Figure 5].
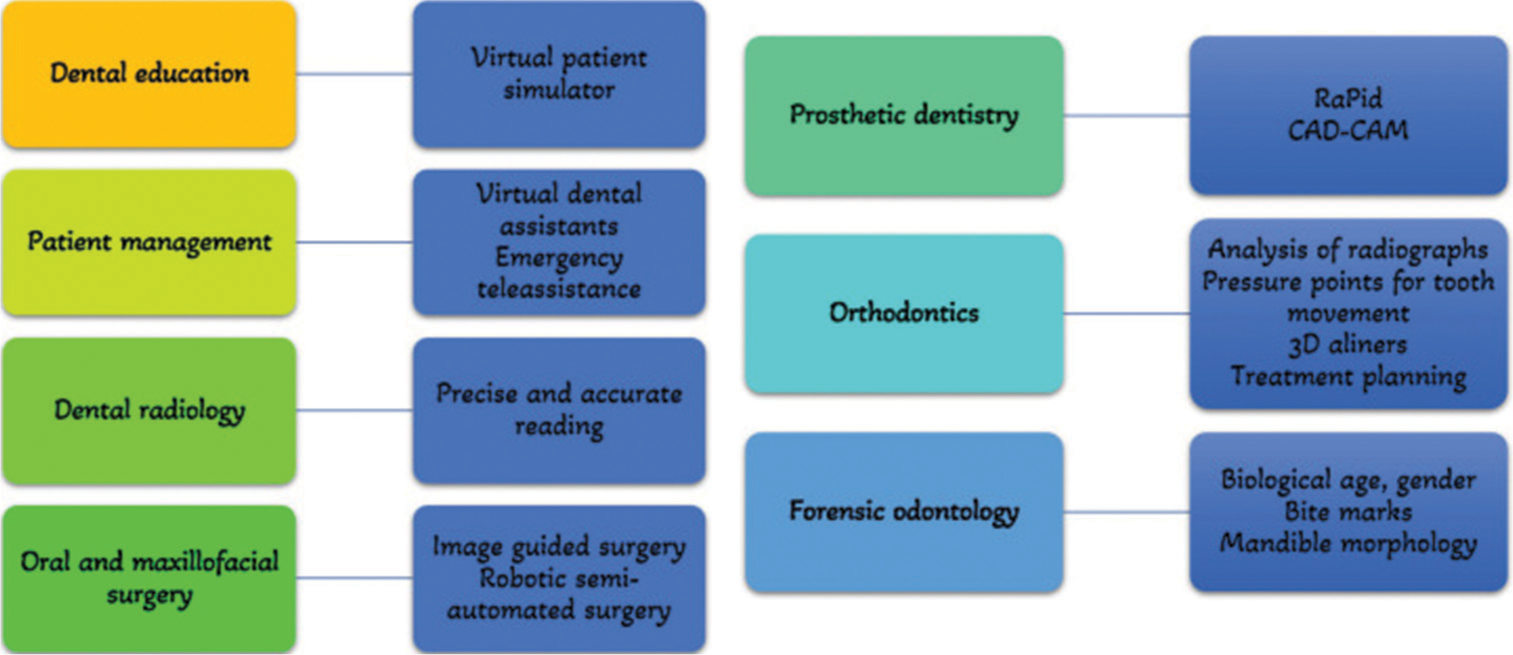
- Artificial intelligence in dentistry. CAD: Computer-Aided Design and CAM: Computer-Aided Manufacturing
AI APPLIED IN ORTHODONTICS
AI plays a significant role across various industries, including orthodontics, where it has become increasingly prominent for precise diagnosis and efficient treatment management.
AI USED FOR LANDMARK IDENTIFICATION AND CEPHALOMETRIC ANALYSIS
Cephalometric analysis is a helpful diagnostic method that helps identify facial disharmonies so that therapy may be centralized and facial growth can be modified. It also helps anticipate facial type and growth pattern.
Automating cephalometric analysis has been attempted in an attempt to decrease the amount of time needed to acquire an analysis, enhance the precision of landmark recognition, and decrease errors resulting from the subjectivity of clinicians. A digital or scanned cephalometric radiograph is loaded by software and stored in the computer during an automated cephalometric analysis. After that, the program locates the landmarks and takes the measurements needed for the cephalometric analysis automatically. Cohen attempted automated cephalogram landmarking for the first time in 1984.[7]
AI is widely used for a variety of tasks, including segmenting teeth, evaluating facial features, determining bone age, forecasting the results of orthognathic surgery, and finding and analyzing cephalometric points. Orthodontic diagnosis is an intensive procedure that involves a patient’s dynamic examination, the evaluation and interpretation of images and radiographic recordings, and model studies. This intricate assessment procedure among orthodontists may lead to various treatment strategies. CephX, WebCeph, AutoCAD software Dolphin Imaging, Dentofacial Planner, Quick Ceph, and AudaxCeph are some web-based software. The AI-based program Automatic Cephalon-Diagnostic Solutions does automatic landmark detection, tracing, measurements, and analysis of cephalometric data [Figure 6].
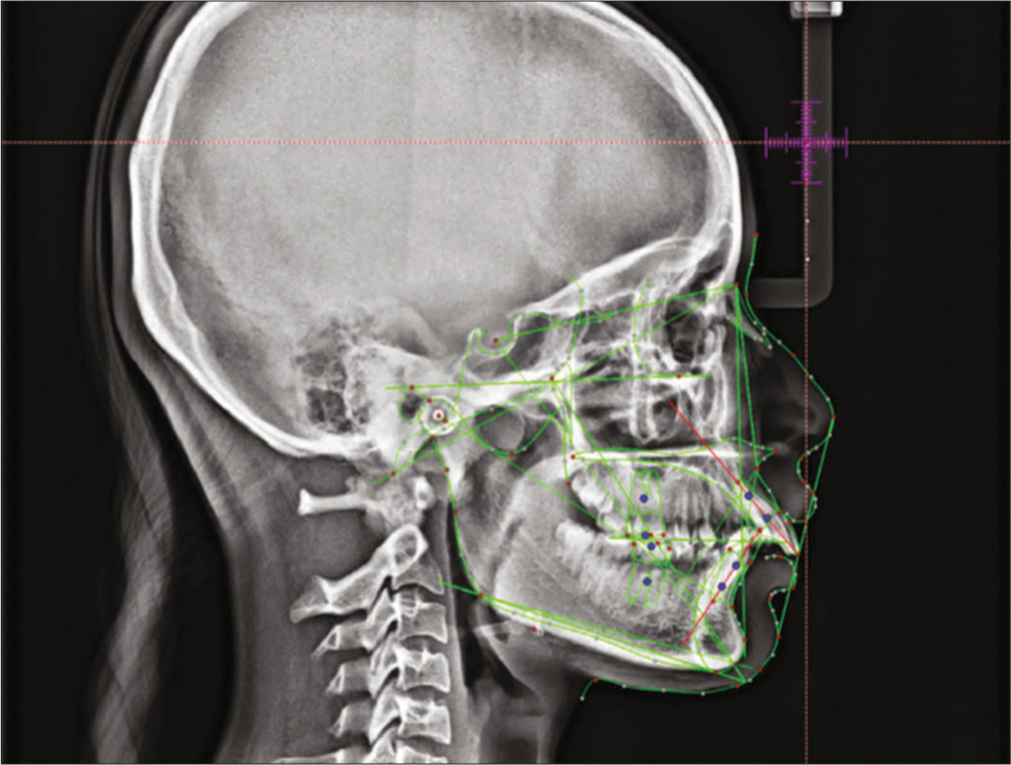
- Artificial intelligence in landmark identification. Green lines indicate tracing of skeletal, dental and soft tissue structures.
AI AND IMPACTED CANINE
An impacted maxillary canine can be predicted in part using angular and linear measurements from panoramic and lateral cephalometric radiographs. Because it provides volumetric estimates of canine occupancy, cone beam computed tomography (CBCT) is essential to the pre-operative assessment. It also helps to evaluate the course for canine eruption guided by orthodontics. Efficient treatment planning and precise diagnosis are guaranteed by this thorough analysis.
In 2010, Nieri et al. conducted a thorough evaluation of the surgical-orthodontic treatment of maxillary impacted canines. They used Bayesian networks to analyze the relative roles and potential causal relationships among key factors influencing the clinical approach. This method provided a comprehensive understanding of how various parameters affect treatment outcomes.[8] Chen et al. employed a machine learning system to assess anatomical differences in the maxilla in cases of unilateral canine impaction. This approach enhanced the understanding and evaluation of maxillary variations in such cases[9] [Figure 7].

- Artificial intelligence and impacted canine.
AI AND GROWTH PATTERNS
In the area of orthodontics, treatment planning is primarily based on how each patient’s unique growth is classified. In research by Lux et al., the growth of 43 children without orthodontic treatment was examined using lateral cephalograms.[10] This work proposed the use of an ANN. For the study and classification of growth-related skeletal changes in general, the morphometric methods used along with the neural network visualization of the growth data thereafter can be utilized.
Cephalometric measurements were utilized in a study by Lakkshmanan et al. to categorize patients’ craniofacial growth as either usual or unusual.[11] The results demonstrated that machines could categorize aberrant development patterns properly 99.8% of the time.
AI AND GROWTH PREDICTION
Timing is one of the most crucial aspects of orthodontic evaluation and plan of treatment.
To computerize the age assessment process using hand and wrist radiographs, machine learning algorithms and AI technologies are being applied. AI systems are capable of deep learning to analyze radiographs after receiving input from a large database that includes information on gender and race. Findings demonstrate that AI systems can assess bone maturity with a level of performance comparable to that of radiologists.[12] Hence, to determine developmental growth, skeletal maturity indices are used. Hand-wrist maturation stages and cervical maturity index are commonly used. In 2010, De Paula Caldas et al. used a computerized and semiautomated technique to measure cephalometric radiographs to estimate bone age.[13] They marked the vertebral points on the computerized cephalometry program and factored in the formula they had formerly developed. Spampinato et al. evaluated bone age using hand-wrist radiographs using deep learning techniques.[14] As a result, more impartial and accurate decisions can be made.
AI AND FACIAL PROPORTIONS
Measuring ratios and the linear distances between facial components are two methods used to evaluate facial proportions. The linear measurements have long been evaluated using profile photographs and lateral cephalometric radiographs, although sensitive measurements are challenging to take due to variations in magnification.
At present, AI applications perform optical facial recognition and simulate complex cognitive functions, including analyzing and interpreting facial data. Research in this field indicates that AI systems could serve as valuable tools in establishing a reliable framework for understanding how individuals sense facial attractiveness.[15]
AI IN DIAGNOSIS OF GENETIC SYNDROMES
AI has been gradually included into diagnosis to increase sensitivity – the ability to correctly predict the presence of an illness or problem in a patient – and specificity – the ability to rule out the sickness or problem when a person does not have it. More than 8000 genetic syndromes have been found. Nevertheless, even with all of the genetics’ advancements, such as next-generation sequencing-based testing, making the right diagnosis is still challenging. Genetic disorders should be diagnosed as soon as possible to optimize results. There is often a significant deal of orthodontic interest in these morphological alterations to the face. Orthodontics is required to address malocclusions and dentofacial deformities caused by a variety of syndromes.
One innovation is the Face2Gene app for smartphones (FDNA, Boston, USA). To identify the tiny patterns that various disorders frequently have, the application compares the image of a patient to hundreds of other photographs stored in its databases. The diagnosis created by the application has proven to be helpful for Asian and Caucasian populations, outperforming experts in the diagnosis of several syndromes[16] [Figure 8].

- Artificial intelligence and syndromes.
IN EXTRACTION TREATMENT
The famous debate of Angle versus Case about whether to extract or not makes it difficult to plan treatment, as it can significantly impact the overall success and stability of the orthodontic outcome. Using a back propagation ANN model, Xie et al. developed a decision-making expert system for the orthodontic treatment of patients between the ages of 11 and 15 to determine whether tooth extraction is necessary.[17] It was decided to treat 120 of the 200 participants with extraction therapy and the remaining 80 with non-extraction therapy. For each patient, 23 indices were chosen as the input data, and the results of extraction or non-extraction were determined. In this investigation, the built-in ANN demonstrated 80% accuracy in the testing set. In addition, IMPA (L1-MP) and lip incompetence were the two indices that contributed the most to the output data. Another neural network model with backpropagation algorithm was created by Jung and Kim[18] The study’s goal was to build an AI expert system for selecting the best extraction therapy and extraction technique. The models had a 93% success rate for determining whether therapy would involve extraction or not and an 84% success rate for choosing an extraction pattern [Figure 9].

- Artificial intelligence in prediction of extraction. (a) Pretreatment photographs and AI predicting the first premolar extraction in lower arch, (b) Post treatment photographs predicting the teeth position after the treatment is completed, (c) Superimposition of pretreatment and post treatment photographs.
AI FOR TOOTH MOVEMENT PLANNING
It seems that orthodontic treatment planning has been assisted by AI for some time now. Many aligner manufacturers claim to optimize orthodontic planning with AI algorithms, saving orthodontist time in the process. AI is a terrific tool to help choose the best way to move a tooth or set of teeth from the pre-treatment position to end treatment position once an orthodontist tells the machine where the end position should be. This is beneficial since bracket-only orthodontics, which is the practice of traditional orthodontics, necessitates a high degree of manual dexterity, which many practitioners lack or have not received the necessary training to develop. These dentists benefit from AI, but machine learning in contemporary aligner therapy has serious disadvantages [Figure 10].
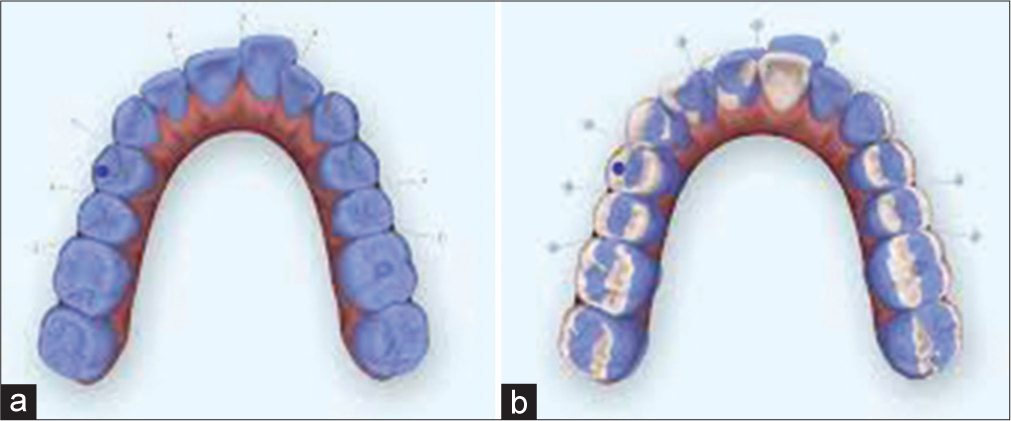
- (a and b) Artificial intelligence and prediction of tooth movement.
PLANNING THE TOOTH MOVEMENT
Insignia by Ormco Customization: AI-driven customization enables the fabrication of personalized brackets and wires tailored to each patient’s dental anatomy. By optimizing the design and placement of orthodontic appliances, AI enhances treatment efficiency and patient comfort while achieving optimal results.
DESIGN OF THE FORCE SYSTEM USING AI
In orthodontic treatments involving extractions, key aspects include individual canine retraction, incisor retraction, and en masse retraction. To achieve optimal clinical and histological outcomes during orthodontic space closure, precise and carefully calibrated orthodontic loops are commonly used to apply controlled forces.
Soft computing approaches, such as AI modeling using neural networks and genetic algorithms, offer advantages in simulating the force systems generated by orthodontic appliances. One significant benefit is their capability to accurately replicate the actual behavior of orthodontic appliances, particularly spring systems.
FORCE SYSTEM PROJECTIONS USING AI
As was already noted, a number of parameters affect the force system of retraction loops, including the force, moment, and moment-to-force ratio. An ANN was created by Kazem et al. utilizing experimental assessments of force systems with T-retraction springs. They conducted an experiment to find how the force system produced by T-retraction springs is influenced by the activation distance and cross-sectional area. Using ANN modeling, they assessed how well their method predicted the force system of T-springs. The network inputs and targets underwent preprocessing procedures to improve the effectiveness of neural network training[19] [Figure 11].

- Artificial intelligence and force system calculation.
ESTIMATES OF TREATMENT OUTCOMES
In dentistry, multiregression models are employed to evaluate the link between a variety of factors and the results. This approach generates a model that represents dependent variables based on their relationship with correlated independent variables, enabling the identification of the most effective predictors.[20] ANNs have been suggested as promising contenders for the prediction of orthodontic results. Kesling’s concept became considerably more workable with the launch of the Invisalign system in 1997, which was the orthodontic treatment method to use digital 3D technology. Invisalign employed a set of algorithms to create changed digital 3D models to make a group of aligner sets rather than needing a fresh model for each stage of the treatment. The technique digitally imitated the teeth moving gradually.
AI software can estimate tooth mobility and the results of orthodontic therapy based on input data and statistical analysis. Comparable expected and actual outcomes are necessary for a valid and successful aligner treatment.[21] AI-based digital systems can also be used for result prediction and teeth control. To validate the software’s simulation capabilities, Buschang et al. compared ClinCheck therapy findings to clinical results once more.[21] They discovered that while the software was effective at replicating simpler treatment plans, the simulation and clinical outcomes in more complex therapies differed noticeably. When it comes to simulating extraction therapy, the ClinCheck program had incredibly low dependability. In complicated cases, ClinCheck models were unable to adequately reflect patients’ eventual occlusion.
ORTHOGNATHIC SURGERIES
Digital orthodontics research and development, as well as 3D orthognathic surgery simulation have received significant funding. In addition, computerized treatment planning and personalized surgical setup design improve diagnosis accuracy. Knoops et al. developed a machine-learning framework for plastic and reconstructive surgery that automates diagnostics and computer-assisted planning.[22] Since this study only used surface scans, it was necessary to compute the underlying bone movement based on soft tissue movement, which is still a difficult process today.
Choi et al. applied ANN using data and concluded that the algorithm had a 96% success rate in determining if a patient needed surgical therapy and a 91% success rate in determining the kind of operation and choice of extraction[23] [Figure 12].
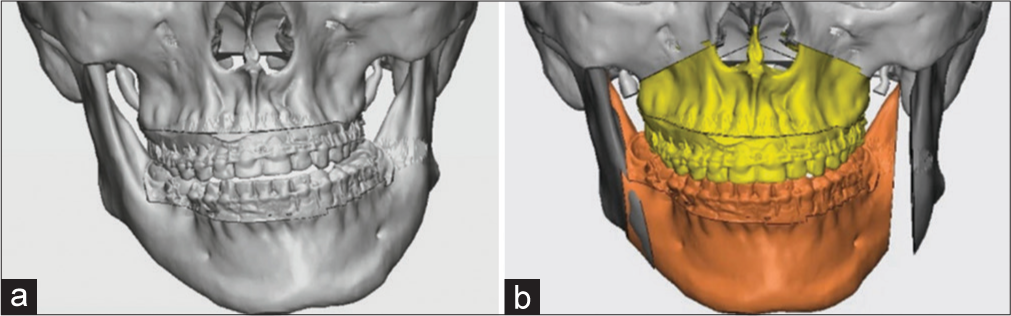
- (a and b) Artificial intelligence and orthognathic surgeries
Thus AI is transforming orthodontics by automating processes, enhancing precision in diagnosis and treatment, and improving patient care [Figure 13].
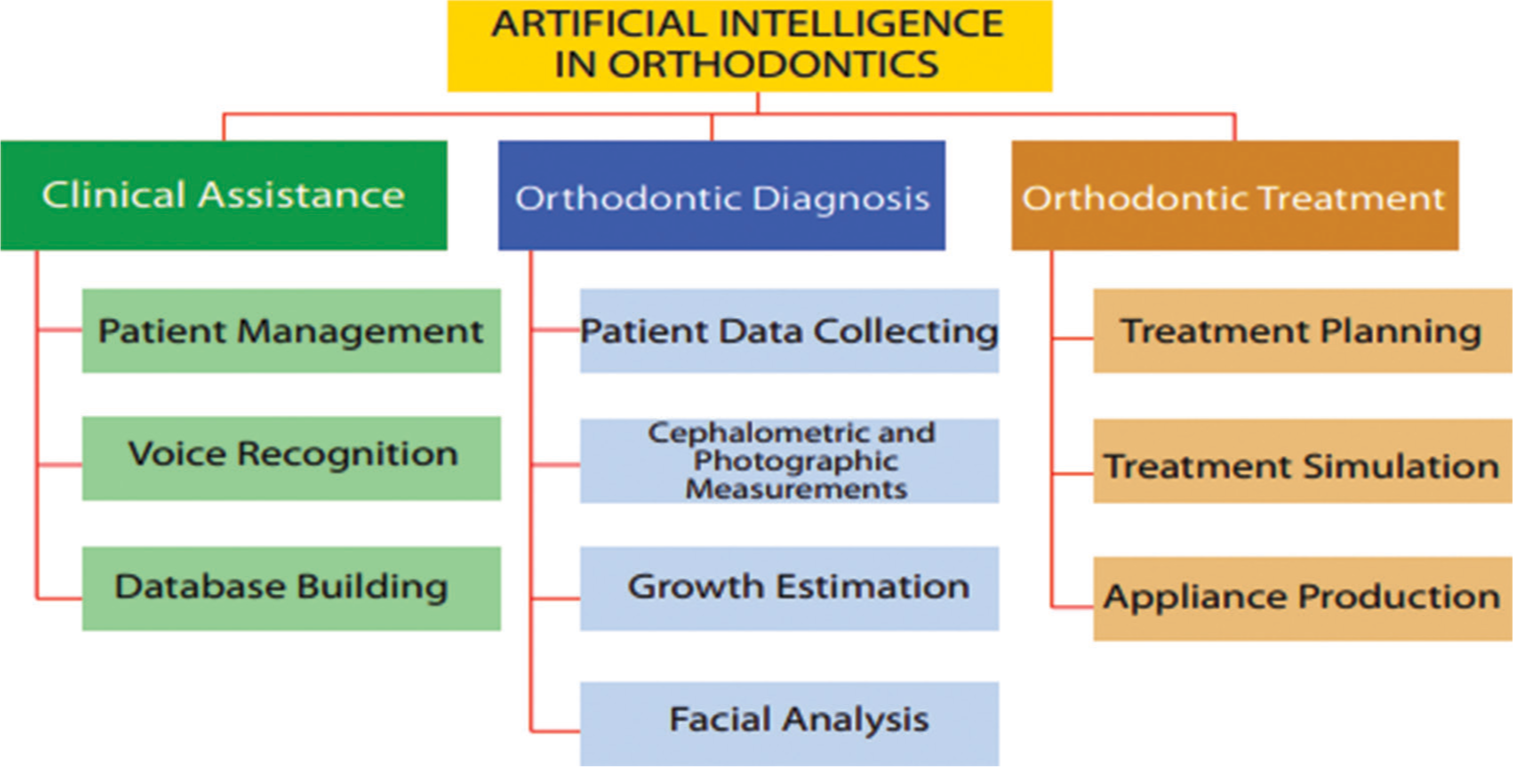
- Artificial intelligence in orthodontics: Summary.
CHALLENGES OF AI IN ORTHODONTICS
There are a number of obstacles and disadvantages to using AI in orthodontics, particularly when businesses offer aligners to patients directly without the necessary dentist supervision. The public has experienced several cases of tooth damage and bone loss as a result of this practice. However, there is a disconnect between the orthodontic community’s professional conferences, where these issues are discussed and the scientific publications where they are published.
CONCLUSION
AI technology is making significant strides in orthodontics, aiding in diagnosis, treatment planning, and prediction of cephalometric landmarks. Despite early limitations, progress is accelerating, promising enhanced patient care through efficiency, accuracy, and time savings. However, AI cannot replace expert clinicians in the near future, as human critical and emotional intelligence remain essential.
Ethical approval
Institutional Review Board approval is not required.
Declaration of patient consent
Patient’s consent is not required as there are no patients in this study.
Conflicts of interest
Dr. Usha Shenoy and Dr. Himija Karia are on the Editorial Board of the Journal.
Use of artificial intelligence (AI)-assisted technology for manuscript preparation
The authors confirm that there was no use of artificial intelligence (AI)-assisted technology for assisting in the writing or editing of the manuscript and no images were manipulated using AI.
Financial support and sponsorship
Nil.
References
- What is artificial intelligence? 2007. Available from: https://jmc.stanford.edu/articles/whatisai/whatisai.pdf [Last accessed on 2024 May 09]
- [Google Scholar]
- Artificial intelligence. 2024. Encyclopedia Britannica. Available from: https://www.britannica.com/technology/artificial-intelligence.24 [Last accessed on 2024 May 12]
- [Google Scholar]
- Comparison of supervised and unsupervised learning algorithms for pattern classification. Int J Adv Res Artif Intell. 2013;2:34-8.
- [CrossRef] [Google Scholar]
- Artificial intelligence: Transforming dentistry today. Indian J Basic Appl Med Res. 2017;6:161-7.
- [Google Scholar]
- Artificial intelligence in dentistry. J Int Clin Dent Res Organ. 2018;10:47.
- [CrossRef] [Google Scholar]
- Application and performance of artificial intelligence technology in forensic odontology-A systematic review. Leg Med (Tokyo). 2021;48:101826.
- [CrossRef] [PubMed] [Google Scholar]
- A preliminary study of computer recognition and identification of skeletal landmarks as a new method of cephalometric analysis. Br J Orthod. 1984;11:143-54.
- [CrossRef] [PubMed] [Google Scholar]
- Factors affecting the clinical approach to impacted maxillary canines: A Bayesian network analysis. Am J Orthod Dentofacial Orthop. 2010;137:755-62.
- [CrossRef] [PubMed] [Google Scholar]
- Machine learning in orthodontics: Introducing a 3D auto-segmentation and auto-landmark finder of CBCT images to assess maxillary constriction in unilateral impacted canine patients. Angle Orthod. 2020;90:77-84.
- [CrossRef] [PubMed] [Google Scholar]
- A neural network approach to the analysis and classification of human craniofacial growth. Growth Dev Aging. 1998;62:95-106.
- [Google Scholar]
- Pattern classification for finding facial growth abnormalities In: Proceedings of the international conference on computational intelligence and computing research. 2013. p. :1-5.
- [CrossRef] [Google Scholar]
- Fully automated deep learning system for bone age assessment. J Digit Imaging. 2017;30:427-41.
- [CrossRef] [PubMed] [Google Scholar]
- Computer-assisted analysis of cervical vertebral bone age using cephalometric radiographs in Brazilian subjects. Braz Oral Res. 2010;24:120-6.
- [CrossRef] [PubMed] [Google Scholar]
- Deep learning for automated skeletal bone age assessment in X-ray images. Med Image Anal. 2017;36:41-51.
- [CrossRef] [PubMed] [Google Scholar]
- Facial attractiveness of cleft patients: A direct comparison between artificial-intelligence-based scoring and conventional rater groups. Eur J Orthod. 2019;41:428-33.
- [CrossRef] [PubMed] [Google Scholar]
- Identifying facial phenotypes of genetic disorders using deep learning. Nat Med. 2019;25:60-4.
- [CrossRef] [PubMed] [Google Scholar]
- Artificial neural network modeling for deciding if extractions are necessary prior to orthodontic treatment. Angle Orthod. 2010;80:262-6.
- [CrossRef] [PubMed] [Google Scholar]
- New approach for the diagnosis of extractions with neural network machine learning. Am J Orthod Dentofacial Orthop. 2016;149:127-33.
- [CrossRef] [PubMed] [Google Scholar]
- Experimental investigation and neural network modeling for force system of retraction T-spring for orthodontic treatment. J Med Devices. 2010;4(2):21001.
- [CrossRef] [Google Scholar]
- An intelligent system for prediction of orthodontic treatment outcome In: 2006 IEEE international joint conference on neural network proceedings. 2006. p. :2702-6.
- [CrossRef] [Google Scholar]
- Predicted and actual end-of-treatment occlusion produced with aligner therapy. Angle Orthod. 2015;85:723-7.
- [CrossRef] [PubMed] [Google Scholar]
- A machine learning framework for automated diagnosis and computer-assisted planning in plastic and reconstructive surgery. Sci Rep. 2019;9:13597.
- [CrossRef] [PubMed] [Google Scholar]
- Artificial intelligent model with neural network machine learning for the diagnosis of orthognathic surgery. J Craniofac Surg. 2019;30:1986-9.
- [CrossRef] [PubMed] [Google Scholar]